New AI deep learning tool detects changes in blood vessels in cancer
Facilitated by the SciLifeLab BioImage Informatics Unit, researchers from Uppsala University, have created HEV-finder, a deep learning AI tool capable of automated detection of morphological changes in blood vessels caused by invasive breast cancer. The tool has been described in the journal of Pathology.
Vascular remodeling, the altering of structure and arrangement in blood vessels, often occurs in the tumor draining lymph nodes (TDNLs), which are the nearest lymph nodes to the tumor itself. Dilation of the so-called high endothelial venules (HEVs), specialized blood vessels which act as recruitment gateways for surrounding lymphocytes, can even occur before the lymph node is “attacked” by cancer cells.
These changes could potentially both contribute to tumor spread (metastasis) into the TDLNs and tumor-induced suppression of the anti-tumor immune response. Interestingly, dilated HEVs can be observed in several types of cancer and have the potential to act as future biomarkers of disease progression and tumor immunity status.
Uppsala University researcher Maria Ulvmar and her colleagues have earlier shown that patients with invasive breast cancer display dilated HEVs. In a recent study, facilitated by the SciLifeLab BioImage Informatics Unit, she demonstrates an automated deep learning AI tool, capable of successfully identifying and classifying HEV dilation in TDLNs of patients with cancer.
Since manual visual assessment of changes in vascular morphology is a tedious and difficult task, only a limited number of patients can be analyzed. This could all change with the new tool, called HEV-finder.
“Our deep-learning tool shows an example of how to successfully approach these urgent needs by high throughput Artificial Intelligence (AI)-based automated analysis. It is designed for analysis of fluorescence images of stained human biobank tissues and can also guide classification of HEVs in very specific areas of the tissue”, Says Tove Bekkhus, first author of the paper.
To foster further development of the tool it is publicly available as an open-source resource and all raw data will be shared.
“The data emphasize that this type of deep learning based tools requires carefully annotated images from manual analysis. To foster further development of these methods we provide HEV-finder as an open-source implementation and make all raw data available as training examples for teaching and development purposes.” says Carolina Wählby, scientific director of the BioImage Informatics Unit.
By using 12,524 manually classified HEVs, the researchers trained a deep learning model and created a graphical user interface for visualization of the results. HEV-finder, selectively analyses HEV dilation in specific regions of the lymph nodes.
“We evaluated HEV-finder’s ability to detect and classify HEV dilation in different types of breast cancer compared to manual annotations. Our results constitute a successful example of large-scale; fully automated and user-independent, image-based quantitative assessment of vascular remodeling in human pathology and lay the ground for future exploration of HEV dilation in TDLNs as a biomarker. My lab will use the HEV-finder for our basic research, to effectively analyze more tumor types, link HEV-dilation to other stromal changes and to changes of the lymphatic vasculature in the cancer patients”, Says Maria Ulvmar, senior scientist who led the study.
The development of the HEV-finder was made possible through close collaboration with the SciLifeLab BioImage Informatics Unit.
“I recommend the SciLifeLab BioImage Informatics Unit (BIIF) for any researcher who would like to develop and expand the boundaries of what is possible for image analysis, and/or who wants to develop solutions that allow us to go from slow manual analysis to high throughput analysis”, Maria concludes.
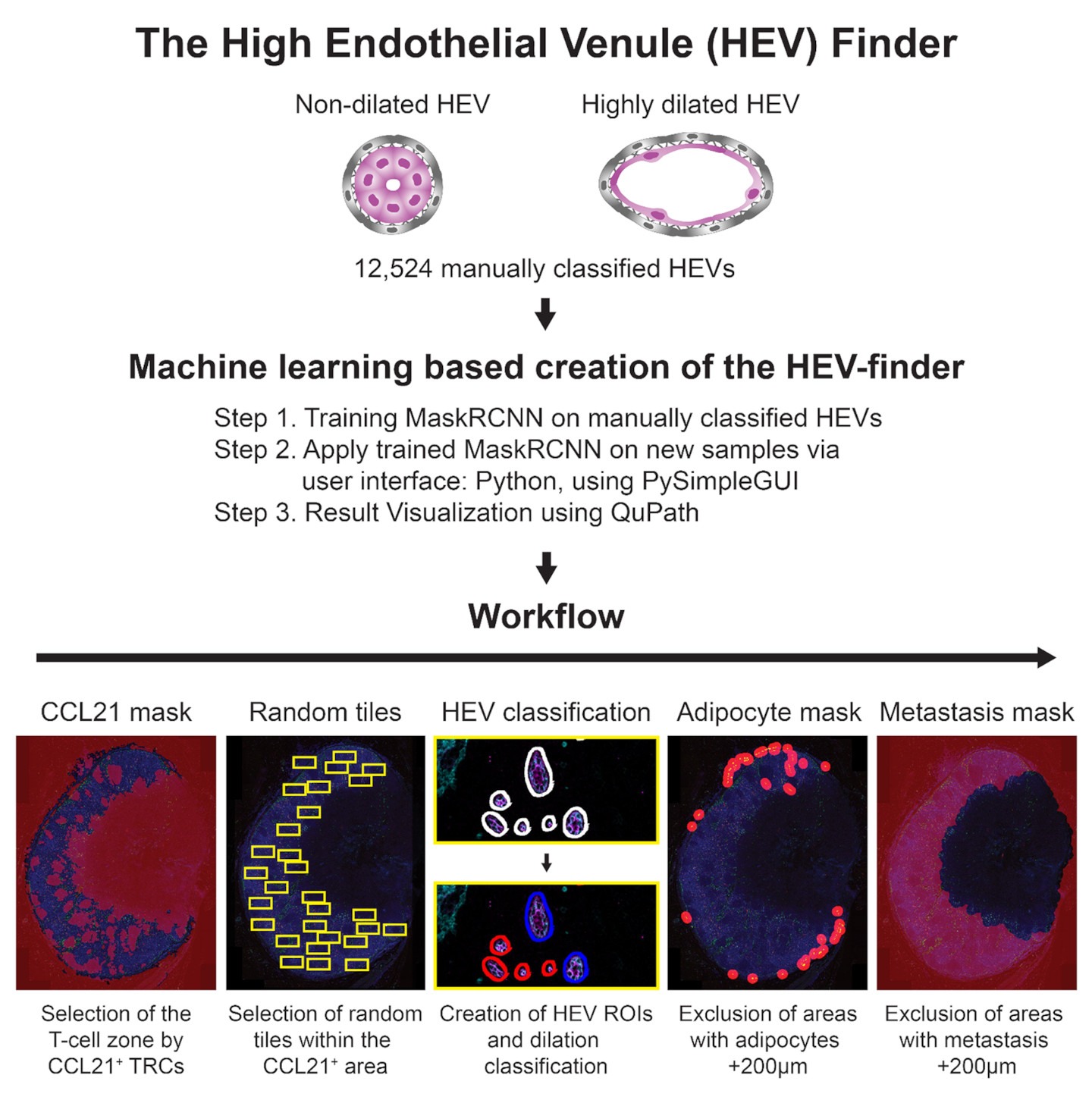