How do conifers adapt under climate change?
Conifers are ecologically dominant and economically important, but are globally succumbing to drought, disease, early-budding and other challenges. One of the oldest lineages on earth, conifers have the genetic diversity to adapt to a broad range of environments. However, long generation times combined with the greatly increased rate of climate change globally challenges trees’ ability to adapt, resulting in weakened individuals and eventually stand loss.
We use quantitative, computational and population genetic approaches in forests across Europe to understand climate adaptation in conifers. To understand how trees adapt to their environment – and how they might perform under changing climate – we first need to understand how much of growth is due to genetics, the experienced environment, and how much is individual variation in environmental response. By focusing on annual growth measured from tree-rings, which form when growth stops during the winter months in temperate regions, we can observe individuals growing across their lifespan. Furthermore, for the years with available environmental data from historical records, weather stations or satellites, we can understand how much of the observed growth was due to the experienced environment. These models provide estimates of how well adapted an individual is to any modeled environment and we have found that they are, in many cases, highly heritable.
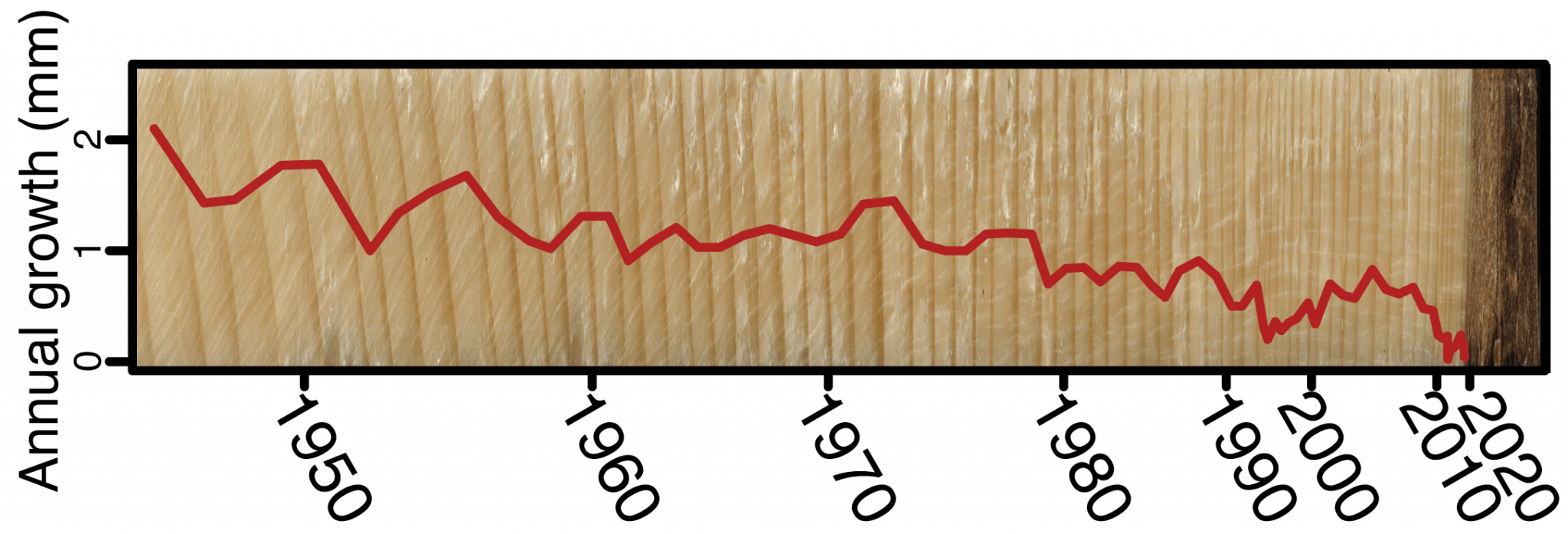
Once we have estimates of adaptation for individuals, we can parse the genome to understand the genetics underlying adaptation, and how this may differ across species and populations. We can also generate predictive models to estimate how well adapted an individual might be to an environment that it hasn’t experienced, validated across populations. Because we are working with tree-rings, we are not limited to controlled populations, providing the means to evaluate any tree’s performance in any modeled environment. As environments shift under climate change, this provides a powerful tool to select parents for healthy, resilient forests.
Group members
Miguel Vallebueno
Miroslav Polacek
Vasilina Akulova
Lucyna Slusarz
Anni Nurmisto
Kirill Akulov
Lisa Wiedlich
Paige Guevarra
Anna Gsteu