DDLS Annual Conference 2023; The emerging role of AI in data-driven life science
November 15 @ 12:30 – November 16 @ 12:30 CET
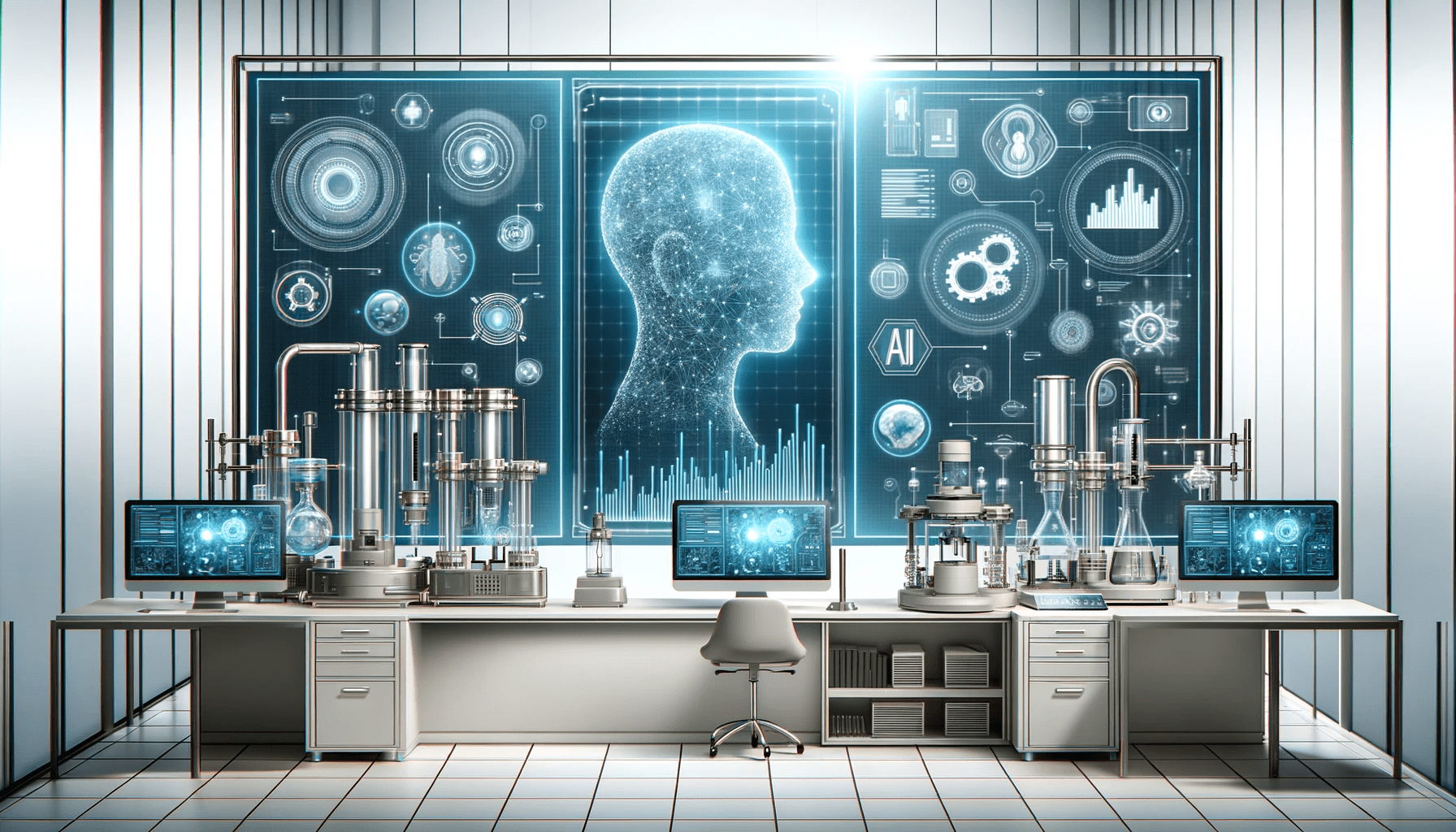
Image generated with DALL-E 3
This DDLS Annual Conference brings together the data-driven community in Life Science, introduces newly appointed DDLS fellows, and provides opportunities for networking across the research community and SciLifeLab infrastructures. The theme for 2023 is “the emerging role of AI in data-driven life science”, and we are excited to welcome Prof. Dr. Fabian Theis, Director of the Computational Health Center and Director of the Institute for Computational Biology and Rocio Mercado, WASP Fellow, Chalmers University of Technology as Keynote speakers. There will be presentations from DDLS fellows, and invited speakers on how they use AI in their research and their future visions. The conference also features two pre-conference introductory lectures on AI for imaging and natural language processing and a post-conference workshop on AI model serving.
Target group: All scientists interested in data-driven research are welcome to join the 2-day lunch-to-lunch Conference in Stockholm.
Registration
The onsite event has reached total capacity. Please register as a Zoom participant to get access to the Zoom link. Feel free to add yourself to the waiting list, and you will be notified by email if a place becomes available.
Poster session
Poster session, Flash Talk & Best Poster Award (click to open)
Poster list
Poster booklet, including abstracts
The DDLS Annual Conference Best Poster Award, encourages the submission and exhibition of high-quality posters carried out by young scientists, including Ph.D. students, post-doctoral researchers, etc. The poster should be on a topic related to data-driven life science. The Prize, which is based upon the decision of a Scientific Committee-appointed Jury, consists of a certificate and a travel grant of up to 5 000 SEK. The travel must be booked and ordered through the DDLS Support team and follow regular University travel policy. The trip should be completed before 2024-12-31.
The Best Poster Award winner for 2022 was Adelina Rabenius, KTH, and we are proud to support her attendance at an EMBO conference this summer.
Organizer: SciLifeLab, host of SciLifeLab & Wallenberg National Program for Data-Driven Life Science, on behalf of the DDLS Steering group.
Pre-conference introductory lectures
In the morning of the first conference day, there will be two introductory lectures; one on AI within image analysis (by Anna Klemm from Bioimage Informatics Facility, SciLifeLab) and one on large-language models (by Magnus Sahlgren from AI Sweden). The purpose of these lectures is to cover the basics and emerging role of AI within these two active fields. No prior knowledge of the fields is required.
Post-conference activities
Note! The Post-conference workshop and different DDLS stakeholder meetings will be handled separately and require separate registrations.
Workshop
Building and sharing machine learning demo applications within life sciences: a practical tutorial. This event is now full, you can register for the wait list only (in case we receive a cancellation). November 16, at 13:30 – 15:30. SciLifeLab Campus Solna, Tomtebodavägen 23. Meet-up in the Lobby. Room Gamma 2 Lunchroom. Participant list here
Stakeholder meetings
DDLS Fellows meeting (DDLS Fellows only). November 16, at 13:30 – 15:30. Biomedicum, Solnavägen 9. Room B0317. Participant list here
DDLS Fellows PhD & Postdocs meeting (for the PhD and Postdoc members of the DDLS Fellows research groups). November 16, at 13:30 – 15:30. Biomedicum, Solnavägen 9. Room B0313. Participant list here
DDLS Cell and Molecular Biology Expert group meeting (for the CMB Expert group and the CMB Data Science Node). November 16, at 15:30 – 17:30. SciLifeLab Campus Solna, Tomtebodavägen 23. Room Earth
DDLS Research area Cell and Molecular Biology Symposium, Nov 17
The DDLS research area, Cell and molecular biology, invites you to the first in-person symposium on November 17 from 9 to 14. The day will be an introduction to the research area Fellows and Expert Group.
Program
November 15
09:30 | Venue: Jacob Berzeliussalen, Berzelius väg 3, Solna Registration is open from 09:30. Hang your poster from 09:30 to 12:30. |
10:00 | Pre-conference introductory lecture: AI in bioimage analysis Anna Klemm, Uppsala University |
10:45 | Pre-conference introductory lecture: Large Language Models Magnus Sahlgren, AI Sweden |
11:30 | Lunch |
12:30 | Welcome Ola Spjuth, chair of Scientific Committee DDLS Annual Conference |
Moderator: Ola Spjuth, Uppsala University | |
12:35 | Data-driven Life Science Olli Kallioniemi, Director SciLifeLab |
13:00 | Blood proteome is a powerful tool to monitor human health and disease Wen Zhong, DDLS Fellow, Linköping University |
13:20 | Artificial intelligence-based computational pathology for precision diagnostics in breast cancer Mattias Rantalainen, KI |
13:40 | Coffee break |
14:10 | Keynote: Generative AI for modeling single-cell state and response Fabian J. Theis, Helmholtz Munich, Technical University of Munich |
15:00 | Short break |
Moderator: Fredrik Johansson, Chalmers | |
15:15 | 5 x Flash Talks à 5 min per selected poster 1. Gonzalo Uribarr 2. Melisa Maidana Capitan 3. Marcel Tarbier 4. Sheng Joevenller 5. Philipp Rentzsch |
15:45 | Poster session with snacks and drinks |
17:30 | End of Day 1 |
17:30 | Speakers Dinner. Invited only. Speakers, DDLS Steering Group, DDLS Fellows, Organizing committee |
November 16
08:30 | Coffee |
Moderator: Laura Carroll, Umeå University | |
09:00 | From super resolution microscopy to modelling: how proteins spatial distribution regulates cellular processes Juliette Griffé, DDLS Fellow, Stockholm University |
09:20 | Multi-omics data integration with robust penalized regression identifies context-dependent signaling networks Cemal Erdem, DDLS Fellow, Umeå University |
09:40 | AICell Lab: Laying the AI Foundation for Data-Driven Whole-Cell Modeling Wei Ouyang, DDLS Fellow, KTH |
10:00 | Data Centre – capabilities and collaborations Johan Rung, SciLifeLab Data centre |
10:20 | Coffee break |
10:45 | Announcement of Best Poster Award |
10:50 | Keynote: Transforming Biomolecular Engineering Through AI Rocio Mercado, WASP Fellow, Chalmers University of Technology |
Moderator: Johan Bengtsson-Palme, Chalmers | |
11:45 | Panel discussion about the emerging role of AI in DDLS |
12:30 | Lunch and end of DDLS Annual Conference |
13:30 | Post-Conference activities (separate registration) Building and sharing machine learning demo applications within life sciences: a practical tutorial. The event is fully booked. SciLifeLab Campus Solna, Tomtebodavägen 23A. Room Gamma lunch room. DDLS Fellows meeting Biomedicum, Solnavägen 9. Room B0317. DDLS Fellows PhD & Postdoc meeting Biomedicum, Solnavägen 9. Room B0313. |
15:30 | End of Post-Conference activities |
15:30 | DDLS Cell and Molecular Biology Expert group meeting SciLifeLab Campus Solna, Tomtebodavägen 23A. Room Earth. |
Abstracts
Pre-Conference Lectures
In this introductory lecture I will present how AI is used in bioimage analysis. The lecture will start with examples of “classical” image analysis to then explain the concepts of machine learning and AI-techniques in bioimage analysis. In the lecture I will give examples of life science projects in which we have trained convolutional neural networks to segment and classify structures of interest in the images, or encode entire images. I will explain why we chose AI-based methods over classical image analysis and will also point to resources of the growing landscape of workflows, pre-trained networks, and repositories of freely available bioimage data + their annotations.
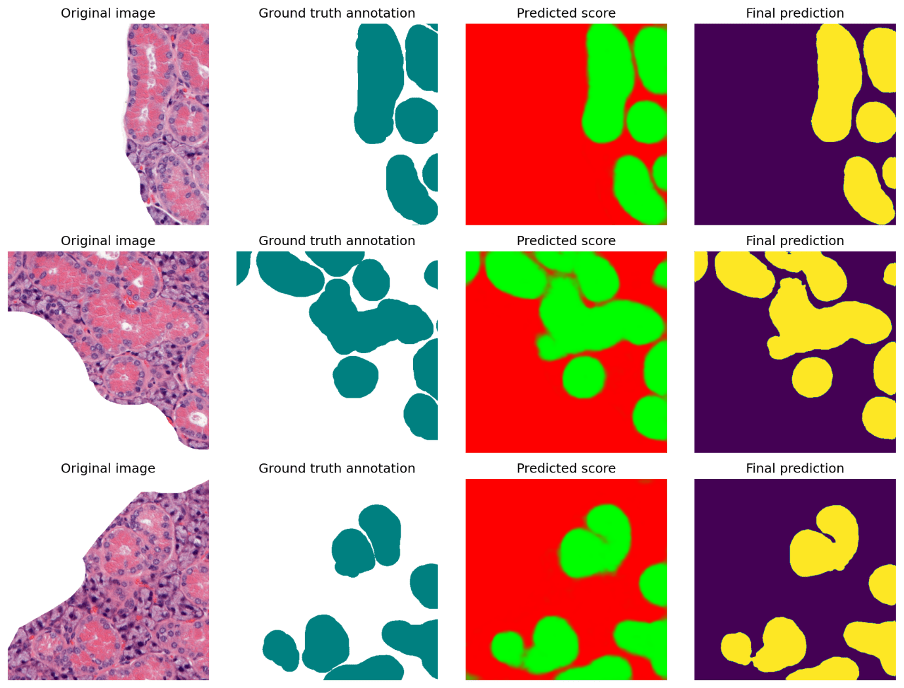
Example of using a CNN for image segmentation on H&E-stained sections of mouse salivary glands. Segmentation starting from manually generated ground-truth, using a Xception network model. Source: https://biifsweden.github.io/projects/2023/08/07/EduardoVillablanca2023-1/, Xenia Tolstoy, Christophe Avenel (BIIF)
This presentation gives an introduction to and overview of the currently dominating trend in AI with Large Language Models (LLMs). We cover the theoretical background, basic architectures, training methodologies, and capabilities of LLMs, spotlighting models like the GPT series, as well as Sweden’s national initiative GPT-SW3. We will touch upon diverse applications of LLMs in both the public and private sectors, and address various considerations and challenges related to LLMs, including biases, transparency, and societal implications.
Conference
Prostate cancer diagnostics and treatment suffer from inefficient use of information for clinical decision making, leading to high rates of overdiagnosis and overtreatment of indolent disease at the same time as prostate cancer is the leading cause of cancer death among men. We will in our presentation discuss how we are systematically trying to develop and clinically implement prediction models and artificial intelligence (AI) systems to address these inefficiencies. We will for example discuss the combination of the Stockholm3 test with MRI to improve prostate cancer diagnostics (Eklund et al. NEJM, 2021; Nordström et al. Lancet Oncology, 2021), and show results from the development of an AI-system for diagnosis and grading of prostate cancer in biopsies (Ström et al. Lancet Oncology, 2020; Bulten et al. Nature Medicine, 2022; Olsson et al. Nature Communications 2022), which we have demonstrated can performs on par with internationally leading uro-pathologist. We will also discuss the link between AI and clinical trials and how clinical trials can be transformed into continuous learning systems, which we will exemplify with the ongoing ProBio trial for improving treatment for men with metastatic prostate cancer (Crippa et al. Trials, 2020; De Laere et al. European Urology Focus, 2022).
Cells integrate signals from different external stimuli to decide their fate, such as growth or death. The “context” of a cell -the extracellular and intracellular environments- dictates the structure of signaling networks that determine such cellular responses. Identifying context-specific edges is vital for predicting and understanding cell decision-making logic. However, robust identification of context-dependent network structures remains a broadly unsolved problem. Here, I will talk about a pipeline we built previously to integrate multi-omics datasets using machine learning algorithms in a central-dogmatic view. Applying this pipeline to publicly available datasets, we showed that it could pinpoint differentially activated pathways: (i) in response to two highly similar sister cytokines in breast epithelial cells and (ii) in breast tumor subtypes. I will conclude my talk with a vignette showing how such knowledge can further be utilized to explore new gene regulatory mechanisms.
Single Molecule Localisation Microscopy (SMLM) is a fluorescence super-resolution microscopy technique which allows to image fluorophores in cells with unprecedented resolution (typically 10-30nm). It produces lists of localisations from which fundamental information about key cellular processes can be extracted. We will focus here on how SMLM data sets can be used as input to design dynamic models on cellular membrane biophysics. We will apply these data-driven models to reliably recapitulate and predict the nanoscale requirements for immune cells activation as well as to control and modify cellular signalling in the context of diseases.
AI’s lightning-paced advancements are ushering in a new era of scientific research, and the realm of molecular engineering stands as a prime beneficiary of this transformation. This talk explores the growing integration of AI in the life sciences, with a primary emphasis on therapeutic discovery, shedding light on the current state-of-the-art for molecular discovery and future prospects. We delve into the evolution of deep generative models and their practical applications in molecular engineering, giving a few key examples of reinforcement learning and synthesizability-constrained design in this domain. Moreover, we share recent progress in challenging application areas, such as targeted protein degradation and synthesis prediction. With adaptive AI models, capable of learning from vast datasets in real time, we can streamline computational and experimental resources, improving efficiency as we search through the complex chemical space. The talk will conclude with an overview of leading developments in AI which have yet to see widespread application in the life sciences, offering new horizons for scientists exploring data-driven life science research. These innovative methodologies hold the potential to reshape conventional practices and bridge the gap between scientific exploration and AI-driven innovation.
As part of the Data-Driven Life Science fellows program at SciLifeLab, AICell Lab is actively laying the groundwork for a future powered by AI in cellular and molecular biology. With a focus on long-term strategic planning, the lab is investing in state-of-the-art automated imaging farms, complete with advanced microscopes, robotic arms, liquid handlers, and automatic incubators.
In addition, AICell Lab is developing specialized AI software to augment real-time microscopic imaging, enable smart acquisition, facilitate robotic control, integrate multi-omics data, and power AI-driven cell simulators, among other functionalities. These preparatory steps align with the lab’s long-term vision to build large-scale, comprehensive models of human cells, utilizing both existing multi-omics datasets and new data generated in-house.
Through this focused preparation and investment, AICell Lab aims to redefine in-silico cellular research and drug discovery, contributing to a unified and systematic understanding of human cellular biology.
Advances in single cell genomics nowadays allow the large scale construction of organ atlases. These can be used to study perturbations such as signaling, drugs or diseases, with large-scale access to state changes on the multi-omic and spatial level. This provides an ideal application area for machine learning methods to understand cellular response. With generative AI revolutionizing many fields of science by allowing researchers to explore uncharted territories, generate novel hypotheses, and simulate complex phenomena, we ask how it has been enabling modeling single cell variation, potentially towards a single cell foundation model.
After reviewing deep generative representation learning approaches to identify the gene expression manifold, I will shortly outline some applications on cell atlas building. Then I will discuss interpretable modeling of perturbations on this manifold, in particular effect of drug responses as well as multiscale readouts such as disease state across patients, and how to learn organism-wide cell type predictors. I will finish with extensions towards temporal and spatial observations.
The rise of high throughput molecular technologies has shed lights on the development of next-generation molecular diagnostics and biomarkers for patient stratifications in the precision medicine era. There is an urgent need to develop new systematic tools to combine multi-omics data and link genotype to phenotype to expand our knowledge of complex traits of human diseases. Our research group mainly focuses on the integration of multi-omics, the interplay between genetics and phenotypes, and the development of data-driven strategies/tools for precision medicine. The aim is to investigate the molecular biomarkers for the estimation of disease risks, early diagnosis of disease, stratification of drug treatment response, disease progression monitoring and the stratification of patients.
Workshop
It is becoming increasingly popular to share machine learning models with the community as web applications with an easy-to-use interface. Users can then adjust parameters or submit their own input and see the predictions generated by the underlying model. This tutorial is aimed at researchers working within life sciences who work with machine learning models but do not have the skills to build applications for web. During the tutorial we will start from a trained model and demonstrate step by step how you can create a graphical user interface for your application, prepare it for deployment, and make it available on the web with a URL. We will demonstrate the use of specific tools which make this process easy and doable within a couple of hours.
The workshop will last for 2 hours with a break in the middle. We have room to accept 30 participants, on the first come first served basis. Those registered after that will be placed on the waiting list.
Note that registration for the workshops is separate from the registration for the conference. Please see the workshop page for information on registration.
Scientific Committee
- Chair: Ola Spjuth, Uppsala University
- Johan Bengtsson-Palme, Chalmers
- Laura Carroll, Umeå University
- Fredrik Johansson, WASP, Chalmers
Project leader
- Erika Erkstam, Operations office
events@SciLifeLab.se