Precision cancer medicine (PCM) seeks to tailor treatments to fit the unique characteristics of each individual case. This involves factors like the cancer’s specific mutations and which tissue it originates from. However, accurately predicting the effects of these properties is difficult due to the complex processes within cells, which can make it challenging to identify effective treatments. To tackle this, we are pioneering deep learning models of the molecular networks of cells, to identify systems-level mechanisms in cancer.
Cancer cells reach a state of uncontrolled growth through complex molecular mechanisms originating from various sources. This involves a range of adaptations such as sustained signaling for cell proliferation, altered energy metabolism, and evasion of attacks by the immune system. These adaptations can arise from both internal factors like mutations or external factors such as a viral infection, or inflammation. Additionally, cancer cells interact with surrounding cells in their microenvironment and exchange molecules that further complicate targeted treatment.
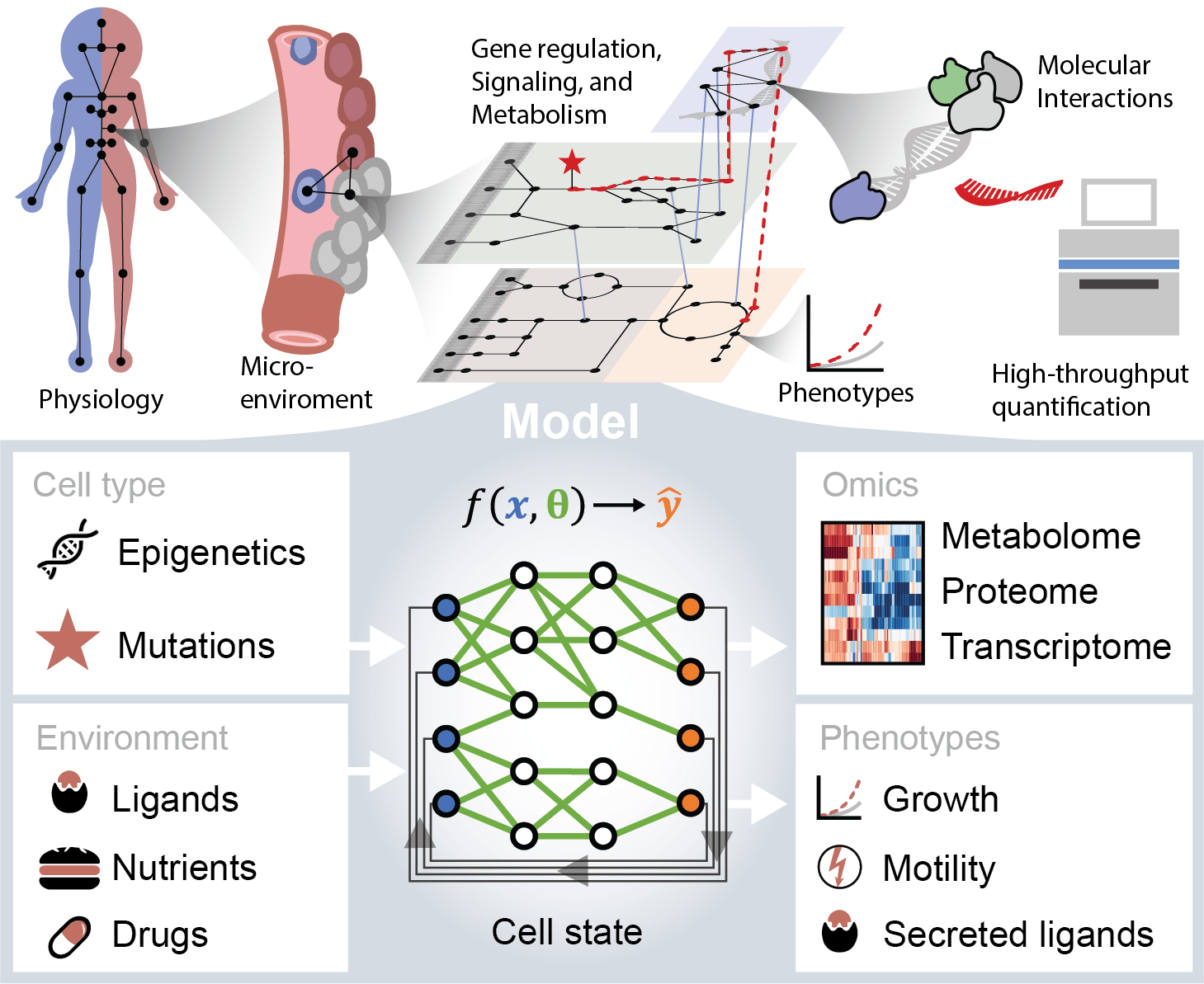
Deep learning models excel at analyzing vast amounts of data to uncover intricate relationships, although interpreting their predictions can be challenging. Our approach is to constrain the models to make predictions that align with the physical interactions between biomolecules, making them more humanly interpretable and actionable. These models encompass various aspects of cell biology, including metabolism, signal transduction, and gene regulation, and are trained on comprehensive datasets spanning many different cell types and conditions, including transcriptomics, metabolomics, and proteomics.
Our objective is to leverage these models to identify novel drug targets, predict resistance mechanisms, and deepen our understanding of interactions between different cell types within the tumor microenvironment, with a specific focus on cancer-stroma interactions in pancreatic cancer. Ultimately, our goal is to facilitate the development of personalized cancer treatments through computer aided design of cancer medicine, thereby improving treatment outcomes and reducing adverse effects for patients.