WASP and DDLS joint research projects
During 2021, WASP and the SciLifeLab and Wallenberg National Program on Data-Driven Life Science (DDLS), the two largest research programs in Sweden, launched a joint call with the aim of solving ground-breaking research questions across their different scientific disciplines. In total, 15 applications were awarded grants for two-year projects.
The call closed on September 1, 2021, but you can read about the call here.
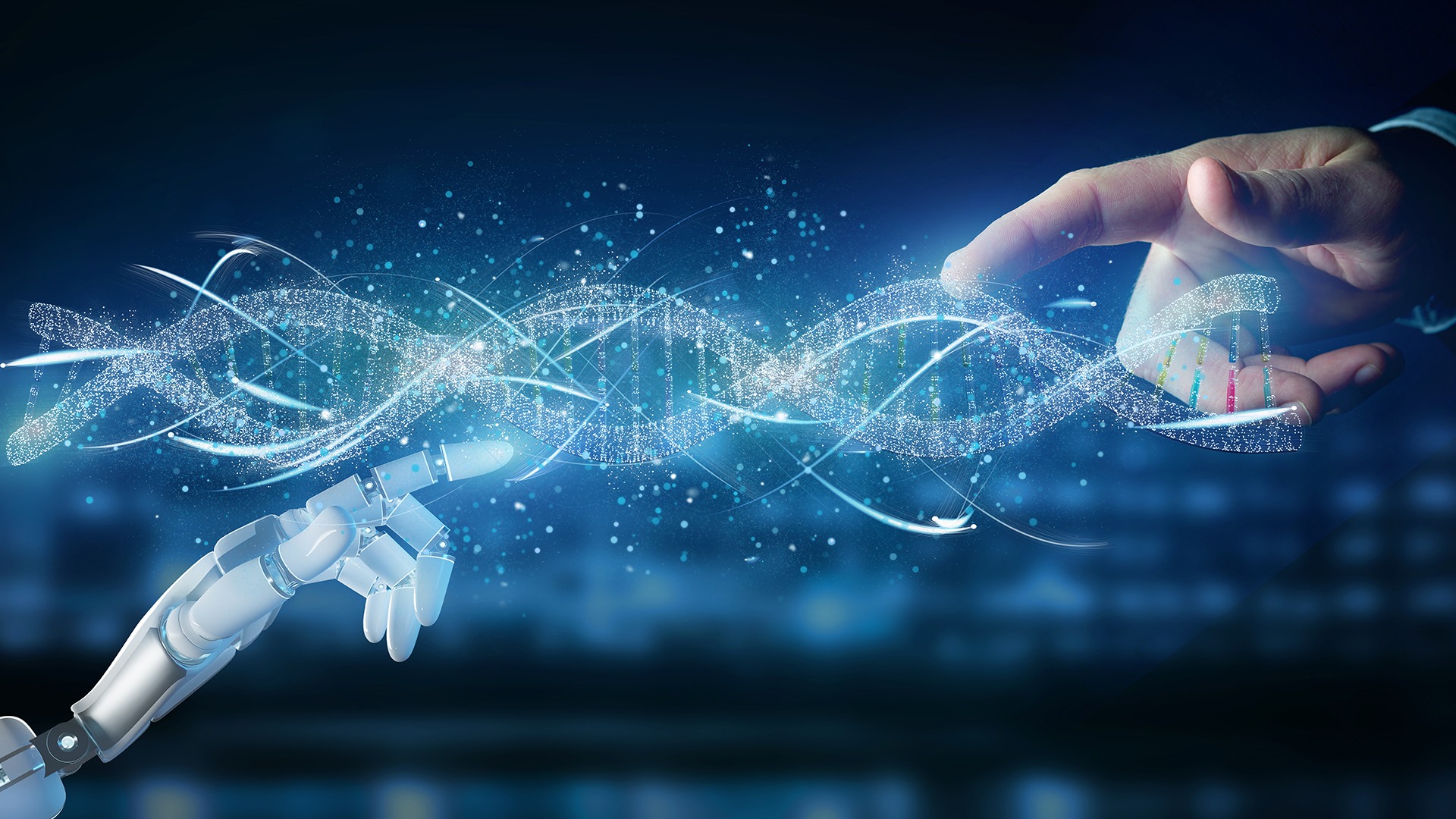
Researchers
Joakim Andén (KTH), Erik Lindahl (SU)
Background
Proteins are essential biomolecules, acting in basically all cellular functions. Single-particle cryogenic electron microscopy (Cryo-EM) has traditionally been the main method for studying the morphology of large protein complexes. Advances in this technique in recent years are now allowing researchers to use cryo-EM to solve near-atomic-resolution macromolecular structures. However, available methods only work for rigid biomolecules and further knowledge about the inherent flexibility and dynamics of biomolecules are essential, not least in drug design.
Aim
To construct data-driven priors of atomic models trained using simulations obtained from molecular dynamics
Methods
Data driven priors in the form of deep neural networks (DNNs) will be used to construct atomic models. The priors will then be used to estimate the posterior distribution of atomic model trajectories given cryo-EM data.
Significance
The project will propose a framework to recover molecular motions and guide multiscale simulations of protein dynamics using cryo-EM data. This would serve as a catalyst for research both in machine learning and structural biology. An important application will be a better prediction of the effects of ligand binding to biomolecules, which is essential in drug design.
Researchers
Arne Elofsson (SU), Hossein Azizpour (KTH)
Background
All cellular functions are basically regulated by interacting proteins. The essential role of proteins in all living organisms are a result of their complex and variable three-dimensional structures, which in turn, enables their different functions. Increased knowledge about protein structures, functions and interactions are therefore fundamental in the understanding of basic cellular functions as well as alterations behind diseases.
In recent years, the AI/deep-learning system AlphaFold2 has radically improved the possibilities to retrieve highly reliable structures of single proteins only from its amino acid sequence. However, predicting protein binding and mechanisms of interaction remains a challenge.
Aim
To develop new deep learning tools to improve predictions of protein binding and interactions.
Methods
A new fold and dock protocol based on improved multiple sequence alignment and the AlphaFold2 tool has been developed, and will be developed further within this project. These tools, along with novel deep learning techniques, will be used to generate models of protein-protein interactions. In addition, the computational power in the AI/deep learning focused Berzelius SuperPOD will be used.
Significance
The tool developed in this project will be used to predict the structures of all known protein-protein interactions in open source databases, and thereby provide the biological community with a large library of protein interaction models. In the long term, this will increase the understanding of cellular functions as well as disease mechanisms.
Researchers
Mika Gustafsson (LiU), Rebecka Jörnsten (GU/CTH)
Background
Many common drugs work ineffectively for sub-groups of patients, as a result of the interplay between a multitude of small-effect genetic and epigenetic factors in complex diseases. New biotechnology methods, called omics, have made it possible to measure molecular imprints of a whole cell, which could be useful for development of more individualized therapies. Deep auto-encoders (DAEs), a type of artificial neural networks, are flexible non-linear dimension reduction methods, that recently have emerged as effective tools for summarizing high-dimensional complex genomics data.
Aim
To create a flexible multi-omic data integration tool that captures disease-specific structure across multiple levels of biological data to help identify processes related to disease outcome, severity, and response to treatment.
Methods
DAEs will be developed with latent spaces constrained by biological side information such as cellular pathways, that can be combined into Deep translational networks (DTNs). The DTNs are further constrained with biological information on the samples, e.g., disease subtypes or clinical outcome.
Significance
More advanced data-driven data-integrative methods will be essential in biology as multi-omics single-cell data sets are rapidly emerging. This project will illustrate how flexible integration of multiple data sources can lead to new insights into disease processes, which is the key to individualized treatment strategies.
Researchers
Joakim Jaldén (KTH), Wei Ouyang (KTH)
Background
Cellular stress responses are shown important in normal human physiology as well as in disease mechanisms. Live cell fluorescence microscopy allows visualization of morphological changes, revealing cellular states. Convolutional Neural Networks (CNN) enable protein pattern recognition and cell segmentation of microscopic images. However, successful training of deep learning models requires capturing not only the major population of cells, but also the minority of rare cells.
Aim
To perform early identification and active tracking of cells with rare cell fates including apoptosis, senescence, and drug resistance, by using reinforcement learning in the live cell imaging setting.
Methods
Two computational elements will be developed:
1) A method that is sensitive enough to identify cell fate groups based on their morphological changes and stress response pattern.
2) A strategy for a self-driving microscope to reduce phototoxicity and maximize the chance to acquire data points for less represented rare cell populations.
Significance
The self-driving system will enable rapid on-the-fly cell fate prediction, automatic microscopy control, and active tracking and acquisition for rare cell populations. The work will provide a framework for modeling the entire cell in a purely data-driven manner. Such digital cell models will further allow in-silico cell experiments which can directly contribute to accelerated drug discovery for a wide range of diseases.
Researchers
Sven Nelander (UU), Rebecka Jörnsten (GU/CTH)
Background
Each year, approximately 1300 new cases of brain tumors are diagnosed in Sweden. A better understanding of the mechanisms behind cellular growth of different tumors are essential to develop new, targeted therapies against the diseases. New biotechnological methods such as single cell sequencing and gene editing show promise as tools to explore brain tumor biology. The data generated by these methods are complex and require new analytical methods.
Aim
To develop a new method to further uncover key genes and cellular pathways behind brain tumor growth.
Methods
CRISPR sequencing will be used for large-scale analysis screening of brain tumor xenografts. Thereafter, AI-models based on neural networks, that integrate and structure the CRISPR-Seq results in relation to other databases will be utilized to reveal therapeutic targets and mechanisms.
Significance
The project will address several challenges including the formulation of a new class of AI models, based on structured and adaptive regularization. The project can increase our understanding of invasive brain tumor growth and the role of AI methods for genomic data interpretation. This will improve our capacity to identify in vivo relevant disease genes, thereby enabling development of new therapies specifically targeting certain tumor diseases.
Researchers
Martin Rosvall (UmU), Beatrice Melin (UmU)
Background
The biobanks and the extensive medical registers in Sweden comprise a goldmine for precision medicine research. However, exploiting the benefits of these resources is limited by the strict regulation of sensitive personal data (GDPR) and the complexity of growing amounts of data.
Aim
Making biobank data accessible by developing new solutions to overcome the obstacles of handling sensitive personal information and analyzing complex data.
Methods
The new biobank infrastructure PREDICT built from the Västerbotten Intervention project will provide data and implement the results coming out from our planned analyses. We will develop a machine-learning approach for data de-identification and evaluation of the de-identified biomedical data based on risk for re-identification and data quality, allowing data sharing and recycling unrestricted by GDPR.
In addition, we will develop methods for fast, interactive biobank data visualization and dimensionality reduction for longitudinal data from repeated samples donated by the same individual over time, enabling a broad research community to access the data.
Significance
Making the biobank data accessible will propel precision medicine research, advance its clinical use, and ultimately improve population health and survival in major endemic diseases.
Researchers
Kevin Smith (KTH), Theodoros Foukakis (KI)
Background
Breast cancer is the most common cancer for women and around 1,500 patients die each year in Sweden due to the disease. Regular mammographic screening has been demonstrated to decrease mortality by 20% to 40%, however, this method has limited sensitivity for some tumor types. ScreenTrust, an AI-based approach, has been developed for estimating breast cancer risk from screening images with some success. However, all models have been based on Convolutional Neural Networks (CNNs) that only accept a single image as input data.
Aim
To develop a new class of neural networks for breast cancer risk assessment that considers information from other views and historic images, to improve early detection and select patients for more sensitive screening methods.
Methods
Vision transformers (ViTs) will be used, which can outperform CNNs on standard vision tasks. ViTs provide a powerful way to combine multiple mammographic views from a patient. In addition, the ViT can treat an individual image or an entire patient’s clinical history as a sentence and learn which words (or regions) to pay attention to for the task at hand.
Significance
Reliable AI-based methods to estimate breast cancer risk offer a potential solution, which could allow hospitals to offer effective personalized screening regimens and care to women, thereby increasing breast cancer survival.
Researchers
Petter Brodin (KI), Dimos Dimarogonas (KTH)
Background
Immune systems in humans vary a lot between individuals. At the same time, the composition of cells in the blood is stable within an individual over time in the absence of perturbations such as an infection. To understand higher order functions in this complex system and its regulatory mechanisms, simultaneous analyses of all cell populations are required. Technological advances now allow such analyses.
Aim
To develop a network model for the immune system responses.
Methods
A novel single-cell genomics approach established in the Brodin lab will be used where all immune cell populations are stimulated and analyzed in whole blood cultures. Cell composition will be modulated with individual cell types depleted and the consequent functional responses by remaining cells analyzed. Using state-space techniques, a network model of different immune system responses will be modelled. This can be used to design strategies on how to improve immunomodulatory therapies, by utilizing and expanding leader-selection and pinning control methodologies.
Significance
Understanding cell-cell dependencies and the regulatory network of immune cells will allow human immune responses to become more predictable. Precise immunomodulatory treatments may be devised using data-driven precision interventions, targeting the most important nodes in the immune cell network of a given patient.
Researchers
Tino Ebbers (LiU), Ingrid Hotz (LiU)
Background
Personalized medicine is at the center of all discussions of future medicine. The promise is to enable earlier diagnoses, better risk assessments, and optimal treatments by providing patient-specific care and treatment. The foundation for this is the generation of realistic patient models, which are largely based on high-quality imaging techniques.
Consequently, medical imaging data is continuously growing in size and complexity, where every generation has resulted in more data per exam. However, it also gives rise to many challenges in terms of handling and accessibility of a huge amount of complex imaging data.
Aim
To provide a pipeline for the next generation of personalized heart models.
Methods
Data will be collected by using the image acquisition technology of the future and methods that support an efficient and effective analysis of the data for diagnosis and model building, integrated in a visual data browser with high-quality rendering, will be developed.
Significance
Expertise from two Postdocs, one with a medical engineering background and the other, with Data analysis and visualization experiences, will be combined in this project. Jointly they will generate detailed heart models, extract flow and muscle strain tensor data harvesting the PCD-CT data. From the generated data, a database according to FAIR principles will be built. The visual data browser will support interactive exploration of the database with high quality rendering of anatomy, blood flow and heart muscle strains, and advanced search function.
Researchers
Mats Karlsson (UU), Bo Bernhardsson (LU)
Background
In the context of precision medicine, the purpose of pharmacometric modeling is to make predictions on an individual basis, based on known possible covariates. Traditionally, covariates have been restricted to demographic parameters (age, weight, etc.), but lifestyle (smoking, exercise, etc.) and omics data (SNPs, etc.) are vastly expanding the possible parameter space, presenting both challenge and opportunity.
Ultimately predictive performance is limited by the amount of available training data, how informative the training data is, and how much of inter-patient variability that can be explained by the covariates present in the data set. One key challenge lies in understanding or learning causality dependences and using them to establish a sound covariate model.
Aim
To develop methods to simultaneously learn the structure and parameters of covariate models and to improve pharmacometric predictors suited for individualized precision medical therapies.
Methods
Two machine-learning methods, Knowledge-based regularization (artificial neural network (ANN) and kernel models) and Learning Low-dimensional mechanistic and casual relations, needs to be developed. Datasets from Propofol, the Diabetes Registry, and the Multiple Sclerosis Registry will be considered for this project.
Significance
Pharmacometric predictions constitute a cornerstone in precision medicine. The existence of well-established national quality registries puts us in an internationally unique position to lead the development of data-driven pharmacometrics modeling, with the prospect of strengthening Sweden’s position within research on the area, and to inspire further collaboration in-line with the objectives of the KAW DDLS initiative.
Researchers
Tuuli Lappalainen (KTH), Bo Wahlberg (KTH)
Background
Understanding causal regulatory networks of the cell is one of the most fundamental gaps in our current biological knowledge. While we know that genomic information is transmitted to cellular functions via complex regulatory networks, we have limited knowledge of how genes regulate each other.
Previous approaches to tackle this question have been unsatisfactory: gene co-expression studies and equivalent correlation-based approaches lack directionality of causation, genetic data is sparse, and classical molecular biology of specific pathways lacks the necessary scale.
Aim
To develop novel computational methods to infer causal regulatory networks in human cells from existing and upcoming CRISPR gene knockdown data sets.
Methods
Modern causal learning models will be adapted to the high-dimensional perturbation data from single-cell genomics experiments to model gene regulatory interactions. The methods will be extended to handle perturbations of thousands of genes, and include uncertainty estimates to distinguish between no causal effect or insufficient data. Single-cell gene perturbation experiments with CRISPR will be used to validate inferred causal networks.
Significance
The combination of novel statistical methods and modern functional genomics toolkit – with scalable CRISPR perturbations coupled with single-cell molecular readouts – provides exciting novel opportunities to infer causal regulatory networks of the cell. Application of these methods to diverse data sets will allow us to characterize basic biology of cellular function, empower design of maximally informative experiments, and discover specific regulatory pathways underlying genetic risk for complex disease.
Researchers
Fredrik Lindsten (LiU), Sebastian Westenhoff (UU)
Background
Determination of biomolecular structures has led to scientific breakthroughs and innovations in molecular biology and new treatments for diseases. The recently developed deep-learning-based system AlphaFold 2 has improved the possibilities for structure prediction of single proteins. However, several challenges remain, including prediction of conformational heterogeneity.
Machine learning models are commonly trained from large datasets, but once trained they are unable to adapt to constraints or auxiliary, instance-specific data during inference. This is particularly problematic when reliable uncertainty quantification regarding their predictions is required.
Aim
To develop novel algorithms to include instance-specific experimental constraints in machine learning models, to bridge the gap between AI predictions and experimental observations, thereby providing new tools for producing reliable, hybrid “predicted/experimental” protein structural ensembles.
Methods
Initially, AI-generated structure predictions for complex of phytochrome proteins will be scored against low-resolution cryo-electron-microscopy (EM) data. Hybrid structures will be obtained, which are expected to significantly increase the resolution compared to the pure data. The solution obtained with experimental constraints will be re-fed into the predictive model. In a second step, probabilistic modeling, and uncertainty quantification (UQ) will be performed to predict conformational heterogeneity.
Significance
The novel hybrid predicted/experimental protein structures will combine the best of cryo EM (high reliability, single particle technique, measures conformational heterogeneity) and predicted protein structures (high resolution, readily available). The novel AI methods are widely applicable, examples include materials design, drug discovery, and personalized medicine.
Researchers
Alexander Schliep (GU/Chalmers), Pär Matsson (Sahlgrenska Academy)
Background
Machine learning (ML) and Artificial Intelligence (AI) have been a remarkable success in improving small molecule drug discovery, from generating novel molecular structures to suggesting synthesis pathways. While small molecules interacting with proteins are the most frequently used drug modality today, alternatives are increasingly explored to address unmet clinical needs. Particularly, oligonucleotide therapeutics – i.e., drugs based on chemically modified short RNA/DNA sequences – are opening new opportunities in disease areas where traditional drugs have failed.
Aim
To predict thermodynamic effects of novel chemical modifications to oligonucleotides, predict the impact of chemical conjugation on the thermodynamics of therapeutic oligonucleotide binding, and enable federated, privacy-preserving learning of thermodynamics prediction.
Methods
Driven by the sample data for thermodynamics of DNA-DNA hybridization, ML models which can serve as the basis for transfer to chemically modified oligonucleotides, will be developed.
Available data for specific chemical modifications will provide input for transfer learning. Extensions to novel modifications, and to combinations of different modification types, will be additionally based on in silico simulations where experimental data is sparse. By using existing data, one of the goals of the project is to predict the impact of conjugation and select suitable conjugates dependent on oligonucleotide sequence and targeted cell type. Finally, competing entities will be allowed to learn ML models for tasks such as off-target binding prediction from pooled data of oligonucleotide binding without compromising privacy of drug candidate data.
Significance
The proposal will greatly expand the speed of exploration of novel individual and combination strategies of chemical modification and conjugations in the design of novel oligonucleotide drugs. The proposal will also suggest a way for pharmaceutical companies and other parties to pool data without sacrificing privacy.
Researchers
Mathias Uhlén (KTH), Andreas Kerren (LiU)
Background
There is a need for a functional genome-wide annotation of the protein-coding genes to get a deeper understanding of mammalian biology. Present genome-wide annotation tools are useful, but require arbitrary cut-offs, commonly obtained via black-box computational models. This may hinder the ability of the analyst to make an informed decision regarding what are relevant fold-changes and detection limits for the underlying transcriptomics data.
Aim
To develop a new data-driven strategy for exploring whole-body co-expression patterns, using interpretable machine learning with the help of interactive visualization techniques, that support informed decisions, leading to better predictions and improved trustworthiness of the results.
Methods
The new data-driven strategy will be based on interpretable unsupervised learning of whole-body co-expression patterns, supported by state-of-the-art visual analytics. Interactive guiding of the clustering process will be used to explore the gene expression landscape in humans and other mammalian species, to create a whole-body map of all protein-coding genes in all major cell types, tissues and organs.
Significance
The new interactive clustering strategy will improve the quality of the classification of all protein-coding genes according to their expression “landscape”, allowing distinct clustering of genes related to tissues and/or functions, such as testis or muscle contraction. Since the data will be publicly available and visualized in the Human Protein Atlas, it will be of world-wide use for the research community.
Images
Researchers
Björn Wallner (LiU), Alexey Amunts (SU)
Background
Protein-protein interactions underlie the dynamic processes of all living cells. Until very recently the main tool to directly visualize those interactions has been cryo-EM. However, this technique is limited to the most stable complexes.
At the moment, the protein structure field are experiencing a revolution with the development of powerful computational structure prediction tools, AlphaFold, which is powered by advanced deep learning neural networks, that can compete with the experimentally obtained crystal structures.
Remarkably, AlphaFold works well for protein complexes even when it is trained on individual proteins. The reason for this is somewhat unclear but protein-protein interactions are not that different from protein interactions within a single chain. However, folding large protein complexes, with multiple linkers, involving transient interactions will still be a challenge.
Aim
To develop new tools to investigate large dynamic multi-component systems.
Methods
AlphaFold will be used to systematically predict protein-protein interactions along the ribosomal assembly pathway, by analyzing previously unpublished high-resolution structures of assembly intermediates of human ribosome assembly in mitochondria. This is done to optimize the AlphaFold pipeline and to provide a proof of principle.
Using previous results, transient interactions and structural dynamics will be explored by retraining the Evoformer and the Structural Module. Finally, a system that is optimized to use raw experimental data or electron densities, in addition to the evolutionary and structural information, will be developed.
Significance
Due to highly flexible nature of many other cellular assembly systems, and the fundamental importance of the biological problem proposed here, the methods pioneered in this project are expected to be visible and therefore applied also beyond the ribosome assembly problem and become a widely used tool for multi-protein macromolecules.